The Turing test stipulates that a computer can be labeled “intelligent” when a person can no longer distinguish whether it is a machine or a human. While most people agree that artificial intelligence (AI) hasn’t reached this threshold yet, the technology’s rapid progress is impossible to ignore. Earlier this year, the New England Journal of Medicine reported that Chat GPT-4 had passed the board residency examination in four of five specialties with a median score higher than the official passing score of 65%.
As AI evolves and is increasingly integrated into clinical care, considering how approaches to health care and clinical practice will change is critical. Three experts tackled this looming topic during the session How Will Artificial Intelligence Change Diabetes Clinical Practice? on Friday, June 21. The session can be viewed on-demand by registered meeting participants on the virtual meeting platform. If you haven’t registered for the 84th Scientific Sessions, register today to access the valuable meeting content through Aug. 26.
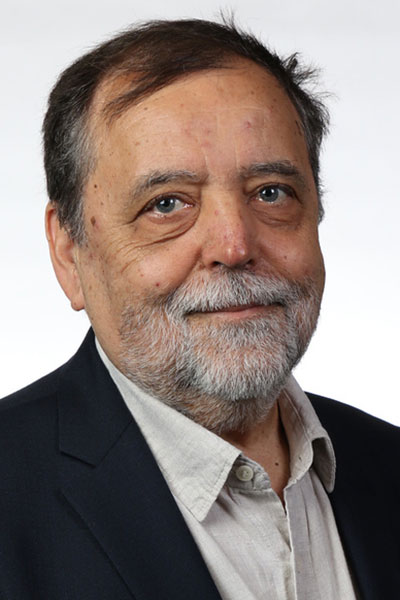
Using AI for automated insulin delivery (AID) is one way to leverage this new technology in diabetes care, and a recent randomized crossover trial using a neural-net artificial pancreas (NAP) has shown promising results, explained said Boris Kovatchev, PhD, University of Virginia. The trial showed that the time in range (TIR) difference between NAP and the University of Virginia Model-Predictive Control (UMPC) algorithm was virtually indistinguishable. Additionally, NAP required significantly lower computational demands than UMPC.
“The neural net [NAP] did exactly as well as the original controller, but it did it six times faster,” Dr. Kovatchev said.
Other emerging techniques that incorporate AI are data farming and AI-augmented clinical trials. The Virtual DCCT Project has successfully recreated the landmark Diabetes Control and Complications Trial (DCCT) in a computer simulation. The results demonstrated that in silico-augmented clinical trials can expand research via biosimulation.
“Studies with infrequent data can be augmented appropriately to generate artificial data that meet the criteria of the study, and it can become larger without spending too much money,” Dr. Kovatchev explained.
More established ways to leverage AI in diabetes care include data science for tasks and research that involve pattern recognition, classification, and prediction.
“Diabetes is the best quantified human disease,” Dr. Kovatchev said. This means scientists can model, virtually simulate, and control the human metabolic system in diabetes with AI.
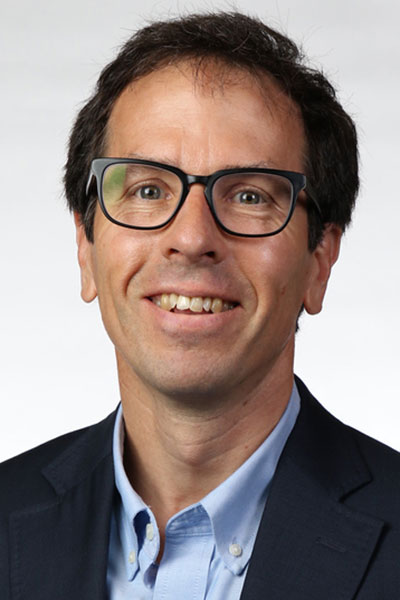
Peter G. Jacobs, PhD, Oregon Health & Science University, envisions automated multi-hormone pumps and AI-enabled smart insulin pens that could detect and forecast future problems, adjust insulin dosing or carb ratios in response to cyclical events, and other advancements that ease the burdens of disease management.
Dr. Jacobs also discussed applications for “digital twins” in metabolic disease research and clinical use. A digital twin is a virtual replica of a physical device, system, or process designed to run simulations to detect and prevent problems, predict performance, and optimize processes.
“What we want to do is physically model metabolism and diabetes and glucose disposal, and all of that, and then replay different therapies to see if you can get better outcomes,” Dr. Jacobs said.
He described how his team used digital twins to train an AI-based k-nearest-neighbors decision support system (KNN-DSS) to share weekly recommendations for people with type 1 diabetes to optimize their carbohydrate ratios, correction factors, and long-acting insulin.
“When people accepted more than half of the recommendations, there was a 6.3 percent increased TIR compared to when they did not accept those recommendations,” Dr. Jacobs said.
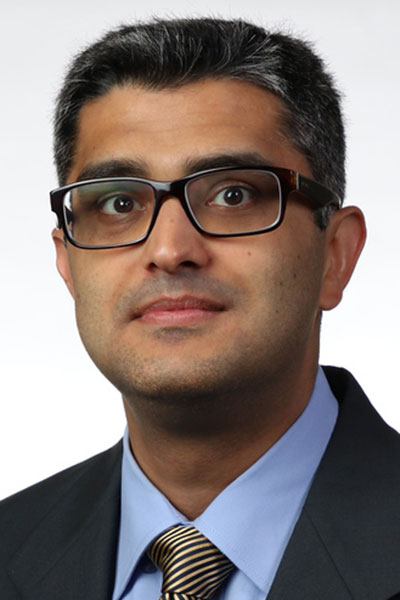
Mudassir M. Rashid, PhD, Illinois Institute of Technology, asserted that AI can help with the efficiency and efficacy of multidisciplinary teams to improve patient care.
“We really need this multifaceted approach in order to treat and care for diabetes, and this multidisciplinary approach is shown to enhance patient outcomes,” Dr. Rashid said.
He highlighted several clinical applications for AI in areas like diabetes risk prediction, treatment selection, and interpreting large volumes of collected data to facilitate multidisciplinary treatment strategies. Dr. Rashid also emphasized the obligation of investigators and those managing patient data to identify and eliminate biases from AI and machine learning datasets while maintaining robust security measures.
“We really need to ensure that there is integrity and security in the data,” he said. “That will help us build trust in these AI systems and mitigate unintended consequences and data privacy concerns.”
Get On-Demand Access to the Scientific Sessions
There is still time to register for on-demand access to learn about the latest advances in diabetes research, prevention, and care presented at the 84th Scientific Sessions. Select session recordings will be available through Aug. 26.